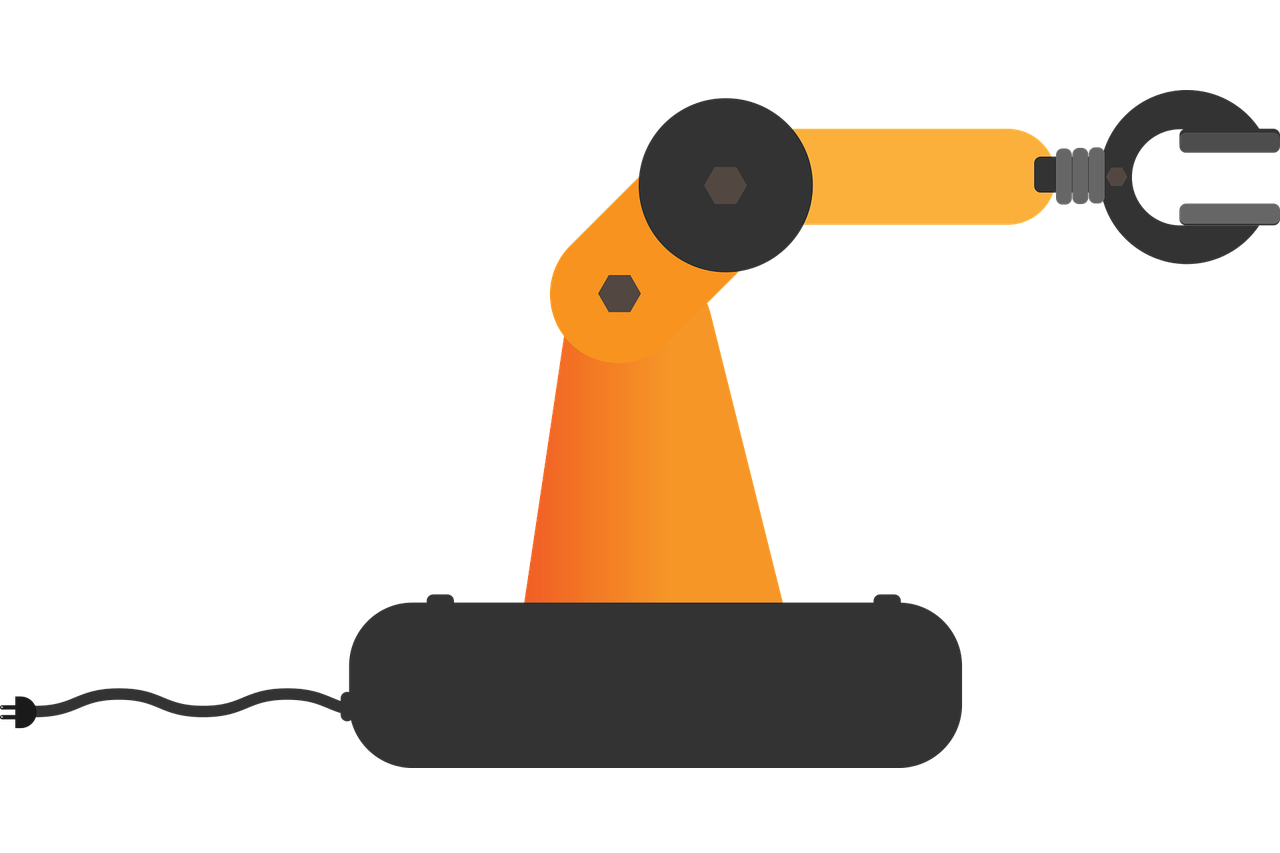
Fund Operator: Why do you feel that the idea that AI will change the fund management industry might be overstated?
Maurice Heffernan: AI technology is evolving, and I keep a close eye on it. It’s probably not overhyped in other verticals, such as the automotive industry with the disruptive nature of automated cars.
On a more generalized basis, it is as big as they make it sound. It’s just not the case in the investment segment of the financial industry.
Neural networks have been around for decades in this business. Application of these technologies has gotten a lot cheaper because of the cloud, the cost of computing, and the amount of data available.
However, I’m not convinced that money was ever the real issue. The plug wasn’t pulled on neural net projects back in the 90s due to a lack of funding, so much as a lack of efficacy. Aspects of AI are not as ubiquitously applicable as some people think.
"Application of these technologies has gotten a lot cheaper because of the cloud, the cost of computing, and the amount of data available"
It’s true that AI has enabled machines to beat humans at chess and GO where there is complete information. AI can now also win at poker, where there is incomplete information and winning requires the human element of bluffing.
However, poker is a fairly narrow domain, where certain strategies get reused. Financial markets, on the other hand, are much more dynamic and are constantly evolving. It is such a fast pace of change within the investment management arena that it takes a lot to keep up.
I do feel that AI has applications in the industry, but I don’t feel that it is as impactful as some might have you believe.
FO: What is the most effective way for the industry to use AI in its current state?
Maurice: Some things are immediately applicable and relatively accessible.
There are different kinds of AI, some which cater more to efficiency plays, whereas others cater more to the alpha-generation objectives. The efficiency play is the more accessible one.
With technologies like natural language processing (NLP) you can get an open source, off-the-shelf algorithm from Microsoft, Amazon or Google in the cloud. You can apply it to a regulatory filing data set to extract, and essentially turn, unstructured data into structured data. You can then incorporate it into a financial model.
This use of AI is fairly accessible these days, and as long as you leverage open source and cloud capability, there aren’t many big barriers.
Where it gets expensive is when you want to move away from that unsupervised learning and into supervised learning. That model involves a training process, which requires a lot of data, subject matter expertise and capital.
"AI is fairly accessible these days, as long as you leverage open source and cloud capability, there aren’t many big barriers"
Conversely, unsupervised learning allows you to do very rapidly filtering and clustering of different data sets in a much more expeditious manner. You don’t have to do a lot of slogging through a rules-engine setup to get at what you’re looking for.
If your organization uses Windows, Azure is very accessible. An organization using Linux might want to go with Amazon. And Google is also fairly accessible and coming on strong, and as they are aggressively playing catch-up they can be easier to work with.
FO: In the unsupervised realm, how accessible is AI to the industry, especially smaller and mid-sized funds?
Maurice: For off-the-shelf, open source algorithms, it is very accessible. We ran an R&D experiment investigating some of the available capabilities last year.
A single individual who was not an AI expert, but was more of a traditional quant developer, was able to learn along the way by embracing the cloud.
"Getting up and running with an unsupervised learning exercise to filter a large data set is fairly straightforward"
A traditional quant developer is typically already familiar with languages such as Python, which tend to be integrated very seamlessly into these near-free cloud offerings.
Getting up and running with an unsupervised learning exercise to filter a large data set is fairly straightforward and can be done by a single individual in a matter of three to six months.
FO: Is that to say that because it is not as complex for people to get on board with the technology that some of the funds who do get the off-the-shelf products aren’t necessarily competing with the larger firms to hire AI technology experts?
Maurice: Yes, I believe so. You need as little as a one- to two-person team to get started, provided you constrain your use case to open source available algorithms.
This includes the industry standard tool set that you get off-the-shelf in the cloud, such as TensorFlow and Python.
For larger players, or hedge fund managers, who want to pursue more complicated alternative data sets and build their own models and algorithms, there is more of a learning curve. It requires an additional investment, where the barriers to entry are larger.
"Large firms will compete for those scarce data-science teams who are in high demand"
Large firms can afford it and will compete for those scarce data-science teams who are in high demand.
You typically find the hedge funds and alt world willing to make these larger investments because the relevancy to their asset class or style of investing, which tends to be shorter term, is higher.
This is in contrast to more traditional, long-only asset managers, who often have more buy-and-hold strategies catering to retirement and other longer-term needs.
FO: Is AI available in cloud-based solutions, and how effective are these platforms?
Maurice: It is evolving quite rapidly, but it does depend on your use case.
Our recent experiments mostly focused on Azure and went very well over the course of the six months that we ran them. Because of the open source influence, API’s and some defacto standards, it is relatively easy to mash up algorithms from Google with a big data environment that you create in Azure, with perhaps an additional algorithm from Amazon.
"If you are going to use something like alt data, the chance of having quick success out of the box is much lower"
Stringing this together is becoming easier. However, depending on the data set that you are working with, unique datasets require contextually relevant algorithms and usually involve some training.
If you are going to use something like alt data, the chance of having quick success out of the box is much lower. Therefore, you are going to have to invest more time, energy, and skill to create proprietary models.
FO: Do you see smaller and mid-sized firms as more nimble when it comes to adopting forward-thinking technology such as AI?
Maurice: As a generalization, your question makes me think of the book The Innovator’s Dilemma, in which frequently the larger player is not the fast innovator. The most common reason given for this is that the larger players are at risk of cannibalizing their own legacy product and margin.
In the case of AI, they have the opportunity to use the technology to make an equally good or better investment decision. This isn’t really cannibalizing their current state, but it does compete with internal traditional value propositions.
"Many smaller players have that start-up mentality where failing fast and cheap is considered a positive outcome"
I feel that many of the larger players, despite their heavy investment, will struggle to keep pace with their more nimble, out-of-the-box thinking small to mid-size players who are perhaps more willing to embrace new technology, take risk, and move quickly.
Many smaller players have that start-up mentality where failing fast and cheap is considered a positive outcome.
I have seen large players incubate successfully internally. I’ve also seen big companies struggle mightily in this process, and it is not for lack of money or talent. Often, it is more about the cultural dynamic.
FO: Does management culture affect how forward-thinking an organization’s approach towards adopting new technology can be?
Maurice: Absolutely. This is a big challenge in a large company. The more layers there are in a firm, the harder it is for the truth to filter up.
It is like a rumor mill at a cocktail party, where every time a story is told, it gets more and more distorted. Similarly, in a large company, if someone has a good idea near the bottom of an organization, as it filters up, it gets distorted.
At the end of the chain, the people who have the authority to make an investment decision have not been given the best information and good ideas frequently experience a premature death.
This article is taken from the research report Fund Technology, Data and Operations, US 2019. To download the full report click here.
Please Sign In or Register to leave a Comment.
SUBSCRIBE
Get the recent popular stories straight into your inbox